When it comes to process development for metal additive manufacturing via laser powder bed fusion (LPBF), the current state of the art looks like this: Print a part, see what you get, adjust process parameters accordingly and repeat.
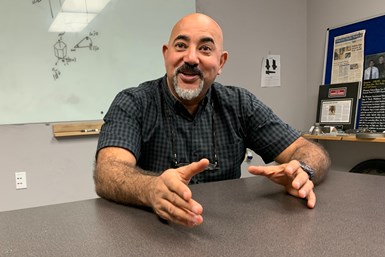
“Microstructure isn't a given, it's an outcome,” says Ayman Salem. His company, Materials Resources LLC, is working to understand the microstructure of 3D printed metal in order to control that microstructure and tailor the material properties of the printed part.
This trial-and-error focuses on geometry. That is, where did the part distort or crack? It also focuses on material properties, with the test build likely to include witness samples for evaluating the performance of the printed metal. When adjustments are needed to improve the outcome in any of these areas, knowing which laser settings or other build parameters to change can come from learned experience, but even the most experienced users of metal AM do not necessarily understand the mechanisms connecting these inputs to the outputs desired.
Ayman Salem, Ph.D., is pursuing this understanding.
He founded a company that is now focused on figuring out these connections between input and output in metal AM. Materials Resources LLC (MRL), based near Dayton, Ohio, is staffed with experts in both materials science and data science. It is equipped with LPBF machines (as well as equipment for other metal AM processes) that are arrayed with custom sensors for process monitoring, which it uses in conjunction with both microscopy systems and an artificial intelligence tool the company has developed internally.
Those mechanisms connecting AM process parameters to the ultimate form and functionality of the part play out at the microscopic level, he says. They happen within the grain or crystal structure of material formation. The effects can be seen, understood and — in many cases — controlled.
Additive Manufacturing Begins With Material Microstructure
Indeed, 3D printing, particularly in metal, is fundamentally a process for making material. 3D printing simply adds the lucky bonus that we get to make material in the shape of a finished or nearly finished part. But the material is what forms first, and getting exactly the material properties and material performance desired is not merely a promise of AM; it is arguably essential for mastering AM’s full capability. According to Salem, the key to controlling LPBF is not learning the knobs to turn within the process to produce the part desired. Rather, the key is knowing what knobs to turn to achieve precise results within the material microstructure — including material phases, crystallography and defects — and even to change these results for different locations in the part. Material properties arise from microstructure, and the functionality of the very form of the part itself arises from this as well.
In other words, get the microstructure right, and everything falls into place.
“Microstructure isn’t a given, it’s an outcome,” Salem says. It is controllable, and in metal AM it is the most important area in which to realize control.
“Microstructure isn’t a given, it’s an outcome,” Salem says. It is controllable, and in metal AM it is the most important area in which to realize control. “To optimize an alloy, optimize the microstructure” — and in this way obtain the properties desired. For example, where wrought titanium Ti6Al4V typically has a tensile strength of less than 1,000 MPa, MRL has demonstrated the ability to manufacture a lower-cost alternative with a tensile strength of 1,400 to 1,700 MPa by manipulating the material’s microstructure. Per Ayman, that specific manipulation relates to “an Fe-containing beta Ti-185 microstructure, and eliminating the beta flecks — that is, no segregation defect — during LPBF.” This adjustment at the micro level yielded a stronger metal.
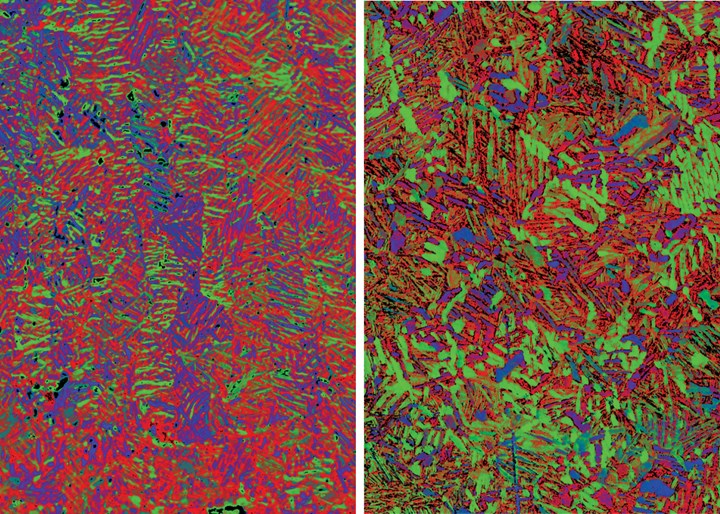
An illustration of improved fatigue strength. Both images show microscopic views of 3D printed Ti6Al4V. On the left is a sample made through traditional laser powder bed fusion followed by hot isostatic pressing (HIP). For the sample on the right, MRL employed LPBF using its own process controls to obtain needed material properties without need for HIP, and also to realize a more useful microstructure. The colors indicate the angular orientation of hexagonal crystals of the metal. The sample on the left is more prone to crack propagation, where the sample on the right can be seen to feature "clumping" of angular orientations that pose a barrier to the spread of cracks. Stopping cracks extends fatigue life, producing a longer-lasting part even though the composition of the metal did not change. Photo Credit: MRL
This is the work to which MRL is committed. The company’s aim is to know, for a given set of desired metal properties, what microstructure will deliver those properties, and how to control AM, including not just the 3D printing but also the feedstock and postprocessing, to attain exactly that microstructure. “We are working on realizing full freedom of material design, not just shape design,” Salem says.
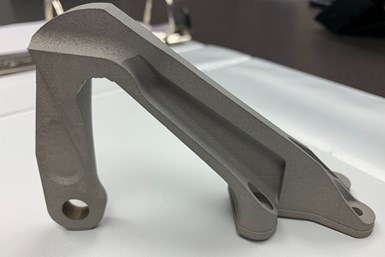
Additive manufacturing offers a unique opportunity to change a part's processing parameters throughout the composition of the part. Because of the thermal influence of different cross sections of a part like this, the parameters may need to be adapted on the fly to realize consistent material properties all throughout the part. Alternatively — and this is the promise particularly interesting to MRL — the part could have different properties in different places. For example, a part like this could be given high fatigue strength around the elbow where stress concentrates combined with high wear resistance at surfaces touching other components, all simply by controlling the additive process for these outcomes as the part is taking shape.
Pursuing this aim has involved a great deal. It has necessitated the invention of a high-throughput microscope for microstructure characterization and computer vision software built on higher-order statistical quantification to recognize and distinguish different microstructure constituents. It also involves physics-based AI tools for analyzing the large volume of potential variables that might be germane to any given material outcome, as well as custom-built, high-speed testing technology for physical specimens so that gathering this information is not the bottleneck that slows understanding. It has also involved retrofitting commercial LPBF machines with enough in-situ sensors so that the machines can, in effect, see, feel and hear the AM process, allowing control systems to steer, adjust and optimize that process as needed.
Topology Optimization and Material Properties
I recently met with Salem to better understand what MRL is learning and how the company is learning it, and to understand how the company will profit from all this — how it justifies the multidisciplinary staff of Ph.D.s this work requires.
In part, the profit is coming from a software resource to be released this year. The company’s ICAAM software (for Integrated Computational Adaptive Additive Manufacturing) analyzes the geometry and intended in-service loading of a part to recommend not just effective 3D printing build parameters along with postprocessing steps, but also the optimal geometry of the part based on MRL’s predictions of the material behavior. Location-specific control of material properties introduces new design options, Salem says, notably topology optimization based on a gradient lattice that changes as needed properties change in different regions of the part.
Location-specific control of material properties introduces new design options, notably topology optimization based on a gradient lattice that changes as needed properties change.
“Topology optimization until now has assumed isotropic material properties,” he says. “We have patented new algorithms to include the inherent anisotropy from LPBF.”
The company’s work also involves prototyping and low-volume production of components in which the part performance requirements entail something beyond a fixed set of global material properties. Fatigue-resistant parts for U.S. Department of Defense are an example. Control over the additive process, and varying the process according to the location within the part and the shape of the part in that area, can yield a final part in which different features have different crystalline structure for different performance characteristics — namely hardness in one region and fatigue resistance only where the part is prone to fatigue. This kind of localized control goes well beyond, and leaves behind, the traditional expectation of fixed material properties throughout the part.
Can We Sequence Metal DNA?
Salem, whose expertise is in materials data science, was not thinking about AM when he founded MRL in 2009. However, he was very much thinking about the potential of better-defined material microstructure. Two samples of the same metal alloy might both contain the same chemical composition, but have different properties because the atoms have arranged themselves into different ensembles of crystal type and crystal shape, as well as volume fractions, phases and grains. The placement of different atoms within cubic or hexagonal crystals of metal affects the ability of those crystals to deform, influencing material strength. The sizes of collections of crystals into grains, along with the preferred crystal orientations within grains, influences strength as well. These are the kinds of variables that might be manipulated through 3D printing.
He says, “The soul of our company has always been the belief that understanding the spatial distribution of atomic arrangements, in its continuous and discontinuous forms, can help scale up our understanding of the product.” That is, the microstructure has a more direct, actionable meaning than we have so far put to use. “I liken it to human DNA. Can we sequence the material DNA?”
“Understanding the spatial distribution of atomic arrangements, in its continuous and discontinuous forms, can help scale up our understanding of the product.”
Much of the company’s work in earlier years focused on the properties achieved in wrought products such as forgings. However, a study aimed at comparing titanium from LPBF to welded titanium opened the founder’s eyes to AM’s potential to not just sequence, but also actively engineer, the material DNA.
The analogy is apt because the work of getting to the understanding Salem describes is vast, ultimately drawing on computation rather than observation. The firm that consisted of just three people in its earlier days studying forgings now includes more than 33 scientists, engineers and technicians focused to a large extent on metal AM. The disciplines within the team include materials science, statistics, data analytics, machine learning, and mechanical, chemical and industrial engineering. Further, the investment in progressively more sophisticated equipment for operations such as X-ray microtomography and electron microscopy ultimately reached the point of MRL needing to invent its own high-speed optical-orientation imaging microscope. Salem says, “Generating spatially resolved crystallographic orientation data at an average of 200,000 pixels per second without the need of vacuum chambers enables us to conduct fast quality control and large-area scans for both additive and conventionally manufactured titanium parts, so that material models and AI capabilities can link processing, microstructure, properties and performance at the part level, not just in simple coupons.”
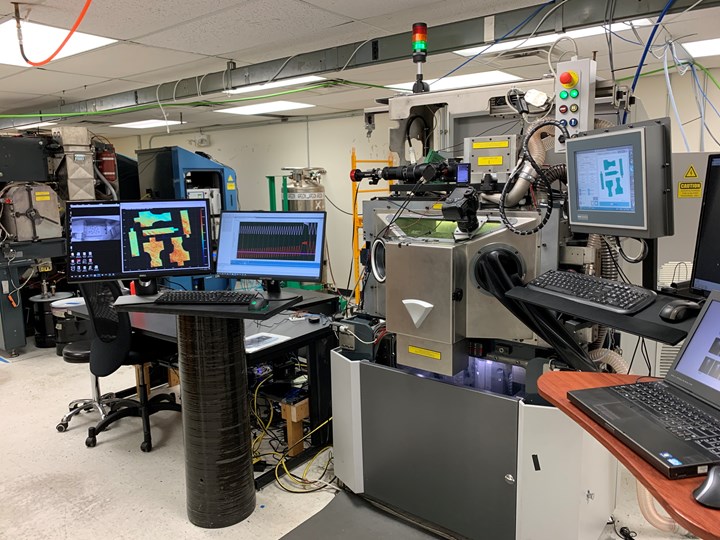
There is a laser powder bed fusion machine to be found under all these modifications. Various visual, vibrational, dimensional, thermal and chemical sensors (most of them unseen in this photo) allow MRL to track variables that are potentially important for the outcome of the build.
The fundamental challenges of the company’s work in evaluating parts relate to speed and scale. What causes changes in AM part microstructure? Answer: a lot. There are a variety of potential inputs at the observable scale — settings of the laser, composition of the raw material, design and setup of the build, and so on — added to the subtle and invisible variability in the condition of the additive machine and its environment — changes in the gas flow, for example — from moment to moment. Meanwhile, there is comparable potential variability in crystal types and grain structure within the same part made of the same material in a single machine. No off-the-shelf hardware system is suited to measuring all the potential variables and no existing software tool was suited to correlating the inputs and effects. On the hardware side, MRL retrofitted its LPBF machines with in-situ and ex-situ sensors far beyond the machines’ standard equipment, adding co-axial meltpool monitors, build layer cameras, acoustic sensors, fume sensors, vibration sensors, a laser for tracking build plate movement, soot detection, temperature gages and more — all to know with precision how process conditions and the material responses are changing moment-to-moment. (The alterations invalidated the OEM’s service coverage on the machines, but the data are worth it, Salem says.)
How to Characterize Additive Manufacturing Microstructure
And an early step, key to making this work, has been the development of a language for numerically defining the spatial information about microstructure. Identifying and quantifying features at a few microns of resolution then can be done using AI tools based on computer vision, pattern recognition, feature extraction and spatial quantification using higher-order statistics, says Salem.
Meanwhile, to link microstructure to part properties economically at a large scale, MRL also developed a high-throughput fatigue testing machine able to be deployed in batteries for evaluating many samples at once.
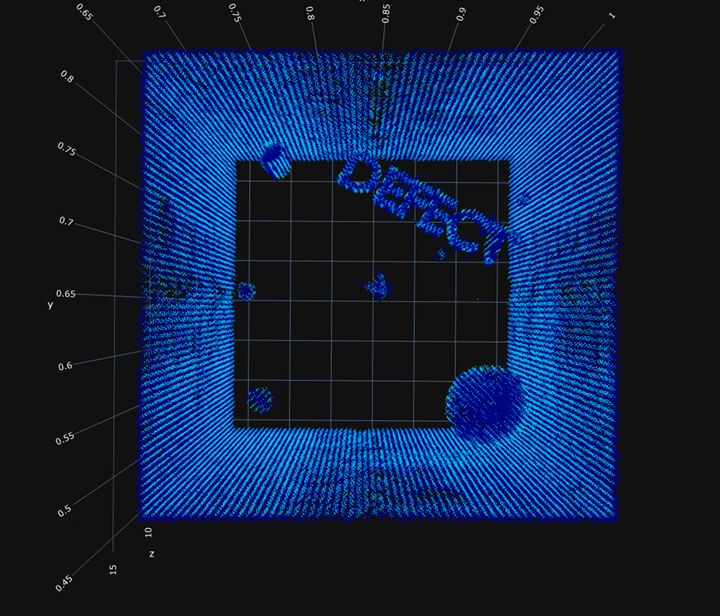
An example of the possibility for precision process control. This is a model created not through CAD geometry, but through thermal mapping of the build as it was in progress. In the build for a 1-cm cube, MRL used control over the laser to engineer deliberate lack-of-fusion zones into the otherwise solid part. The internal “defects” created this way include various simple geometric shapes and the letters of the word “defect.” The thermal map shows how closely the process matched the intended model. Photo Credit: MRL
All this offers a picture of the kind of investment and effort that are warranted to realize the full promise of what metal AM might deliver. That promise is to engineer and finely direct the metal properties to achieve, for instance, rapid and cost-saving 3D printing throughout much of the bulk of the part, combined with 3D printing for a factor-two improvement in fatigue strength only for the region of the part in need of this strength. No other part-making process offers a level of material control like this, and in various alloys so far — aluminum, titanium, magnesium, nickel, cobalt and iron — MRL is delivering on that promise.
Software Instead of Handbook
Artificial intelligence is one of the keys to this success — in this case used in conjunction with integrated computational materials engineering (ICME). Manufacturers applying AM often still imagine it in the frame of other part-making processes, and this leads them to expect that a process like LPBF will one day have a handbook for effective application. But the scope of choices and parameters is too great for this. Instead, MRL’s newly launched ICAAM software likely offers something like the engineering tool that AM will require. In equal parts interactive material handbook, design aid and CAM software, this resource allows the user to import a CAD model and specify load requirements as a necessary condition for finding the right recipe for the build. For the metals that MRL’s AI-enabled analysis has so far explored, the software can use this input to recommend build orientation, laser parameters (apt to vary throughout the build) and postprocessing steps, then predict the resulting part-level strength and fatigue life.
Manufacturers imagining AM in the frame of other processes expect that a process like LPBF will one day have a handbook. But the scope of choices and parameters is too great for this.
The result is a way to set AM free of trial and error. The ICAAM software thus opens the way to the full nuance of what a precisely controllable process like LPBF can do. (And, coming, what directed energy deposition and electron beam melting can do. MRL is training its AI model on these processes, too.) The aim is not just a successful and consistent build, but a part engineered for performance in ways no other manufacturing process could make possible.
At the same time, the software sets AM organizations free of the knowledge that has been developed and applied through human trial and error, and this freedom is no less transformative. Human knowledge is tribal knowledge, and membership in any group is prone to change. Indeed, the current state of AM is characterized by this; talented people with process engineering knowledge in AM might change jobs frequently, and organizational knowledge is limited by the AM experts currently within an organization’s sphere. A tool employing AI is not just a way to advance process knowledge more quickly, and to apply that knowledge more subtly, but it is also a way to secure and systemize the knowledge that has been won, advancing AM more broadly by making process knowledge more universally available.
Related Content
Siemens’ Engineering HEEDS AI Simulation Predictor Optimizes Additive Manufacturing
The HEEDS AI Simulation Predictor empowers organizations to take full advantage of the digital twin to optimize products through advanced state-of-the-art artificial intelligence with built-in accuracy awareness.
Read More5 Observations From Dr. Tim Simpson About the State of Additive Manufacturing So Far
The outgoing co-director of Penn State’s CIMP-3D takes stock of how far AM has come, aided in no small part through the work of the organization he helped to lead.
Read MoreEOS Integrates 1000 Kelvin’s AMAIZE AI Co-Pilot Into Software Suite to Accelerate Productivity and Engineering Speed for Additive Manufacturing
The AMAIZE AI “co-pilot” platform is designed to harness cutting-edge AI to streamline design processes, slash engineering costs and accelerate the introduction of new additive manufacturing products.
Read MoreMPI Launches AI Project to Create Material Reuse Management Tool for Metal 3D Printing
MPI is working with Additive Manufacturing Solutions and AMFG to create a versatile, commercial predictive material reuse management tool that will enable additive manufacturing to expand by introducing greater cost efficiencies.
Read MoreRead Next
Material Innovation Is a Powerful Freedom of AM: Here Are Examples
Possibilities that were speculative a few years ago are coming true today. Applications of metal matrix composite provide an example. Recent articles explore the ongoing advance of 3D printed materials.
Read MoreComputer Vision System Automates Characterization of Metal Powder
A computer vision system that has learned to classify metal powders could speed material qualification and advance machine learning in additive manufacturing.
Read MoreHybrid Additive Manufacturing Machine Tools Continue to Make Gains (Includes Video)
The hybrid machine tool is an idea that continues to advance. Two important developments of recent years expand the possibilities for this platform.
Read More